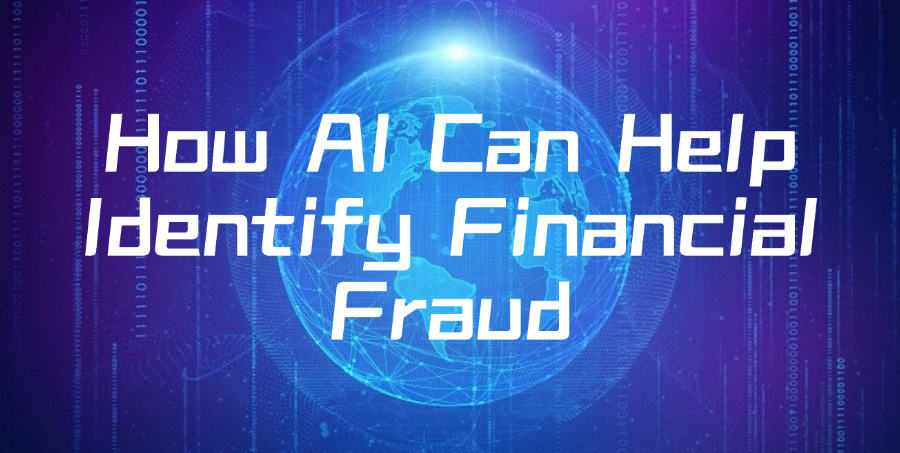
Artificial Intelligence (AI) is revolutionizing financial AI fraud detection and prevention. By leveraging machine learning models, AI can quickly and accurately analyze large volumes of data to identify suspicious transactions and patterns indicative of fraudulent activity. This capability allows financial institutions to process data in real-time, enabling immediate detection and response to potential fraud.
AI-powered Fraud Detection Systems
AI fraud detection systems can process vast amounts of data in real-time, identifying patterns and anomalies that may indicate fraudulent activity. These systems use machine learning algorithms to continuously improve their accuracy and effectiveness over time. Deep learning, a subset of machine learning, involves training neural networks to recognize patterns in data, which has been successfully applied to financial fraud detection.
Key advantages of AI fraud detection systems include:
- Detection of complex fraudulent activities
- Real-time analysis and response
- Improved accuracy and reduced false positives
- Continuous learning and adaptation to new fraud patterns
AI Detection Methods
AI employs various machine learning models to detect fraudulent activities by analyzing patterns and anomalies in data. These methods include:
- Automated anomaly detection: AI algorithms are trained to recognize patterns in data that suggest anomalies, such as unusual transaction amounts, multiple transactions from the same device, or purchases made from different locations in a short time frame.
- Behavioral analysis: This approach involves analyzing customer behavior patterns over time to identify unusual activities. For instance, if a customer suddenly changes their spending habits or makes transactions in unexpected locations, the AI system can flag these activities as potentially fraudulent.
- Natural Language Processing (NLP): NLP algorithms analyze customer communications, such as emails or chat transcripts, to detect deviations from usual patterns. For example, if a customer suddenly changes their account information and sends multiple password reset requests, the AI system can identify this as a potential fraud attempt.
Machine Learning Models in Fraud Detection
Machine learning models, both supervised and unsupervised, play a crucial role in AI fraud detection:
- Supervised learning: Trained on labeled datasets to recognize fraud patterns
- Unsupervised learning: Analyzes data to find hidden patterns without labeled data
- Anomaly detection: Identifies data points that deviate from expected patterns
- Behavioral analysis: Examines customer behavior patterns to detect suspicious changes
Real-Time Analysis and Pattern Recognition
AI excels in processing large volumes of data in real-time, allowing for immediate fraud detection and response. AI algorithms can identify patterns indicating potential fraud, such as:
- Unusual transaction volumes
- Atypical access times
- Multiple transactions from the same device in a short period
- Transactions from unexpected locations
Continuous Improvement of AI Fraud Detection Systems
AI systems improve over time as they are fed more data, enhancing their predictive accuracy and efficiency. This continuous learning process is crucial for adapting to evolving fraud tactics. Generative AI plays a significant role in this improvement by creating synthetic datasets based on real data, which is valuable in scenarios where viable examples are limited.
Benefits of AI in Fraud Detection
- Speed and real-time detection: AI systems can monitor transactions 24/7, ensuring that any fraud is caught as it happens.
- Accuracy and reduced false positives: AI’s data analysis capabilities surpass human abilities, leading to more accurate identification of fraudulent transactions.
- Scalability: As transaction volumes grow, AI systems can expand their monitoring capabilities without proportional increases in staffing.
- Cost savings: Automation of fraud detection tasks leads to more resource-efficient operations.
- Improved risk management: Predictive algorithms can act as a preventative measure, alerting to potential fraud before it occurs.
- Enhanced customer trust and satisfaction: When customers feel secure in their transactions, they are more likely to remain loyal.
Applications in Banking
AI is revolutionizing fraud detection in the banking sector by:
- Continuously monitoring transactions for anomalies
- Analyzing credit and loan applications to detect synthetic identity fraud
- Providing real-time detection of suspicious activities
- Minimizing false positives to enhance customer experience
- Tackling common banking fraud types such as identity theft and credit card fraud
Enhanced Security
AI provides robust security by dynamically adapting to new threats and blocking fraudulent activities in milliseconds. AI systems can process large amounts of data in real-time, identifying patterns and anomalies that may indicate fraudulent activity. This real-time analysis allows for immediate action, preventing potential economic loss.
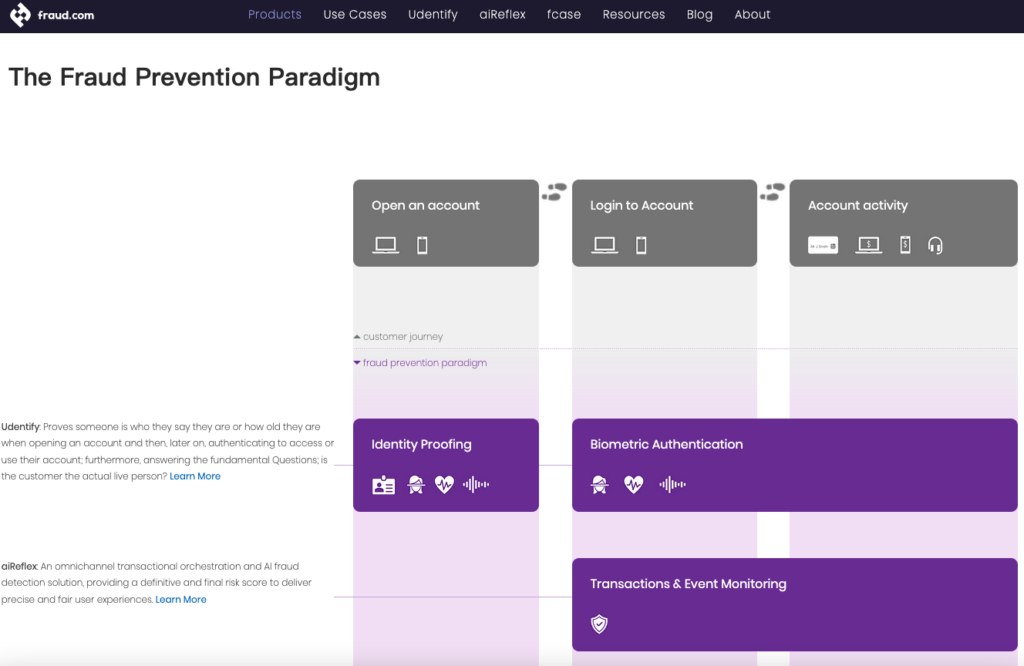
Future of AI in Fraud Detection
The future of AI in fraud detection looks promising, driven by advancements in:
- Generative AI: Offering sophisticated capabilities to identify and anticipate complex fraudulent schemes.
- Integration with blockchain: Providing a secure and transparent ledger for storing transaction data.
- Advancements in Natural Language Processing (NLP): Analyzing unstructured data sources to identify potential fraudsters.
- Enhanced continuous learning capabilities: Making systems more effective at adapting to new fraud patterns.
However, as AI becomes more prevalent in fraud detection, ethical considerations must be addressed. Human oversight is crucial to ensure that AI-powered systems are transparent, explainable, and do not perpetuate biases or discrimination.
In conclusion, the application of AI in fraud detection continues to evolve, providing financial institutions with powerful tools to combat fraud. With ongoing innovation and responsible deployment, AI promises to significantly improve the efficiency and accuracy of fraud detection in the coming years.
Back to Homepage
More:
1.Generative AI for Fraud Detection: Mechanisms & Real-World Examples (masterofcode.com)
2.Identity Verification & End-to-end Customer Onboarding Platform | HyperVerge